At the heart of ARLIS’s work is our expertise in sociotechnical systems - the interaction between people, technology and the decisions they make together. In this article, we’ll explore one key aspect of our sociotechnical expertise that we are applying to solve our nation’s security challenges.
Over the past several decades, the growth of communication tools, information, and technology has changed how humans make decisions. These decisions can vary – some may be immediate or delayed; some based on emotion, logic, or both, and some can even be subconscious. These decisions interact with the actions of others, shaping behaviors and various social structures. They can lead to complex patterns of human behavior and interaction, making it difficult to predict or manage.
For example, we may need to understand how collective behavior can drive economic or technological outcomes, such as how policies or laws shape the U.S. tech landscape. We could also be studying how adversaries may respond to strategic military movements or global political positioning. In some cases, we have even used these insights to plan how to safely evacuate large crowds in emergencies.
This kind of collective behavior is what is called a non-linear complex adaptive system. Non-linear means that a small change can lead to a massive, unexpected result. Predicting this is extremely difficult. Complex means that these systems are made up of many interconnected parts that affect each other. Adaptive means that the system changes its decision-making process over time in response to new information or shifts in the environment.
These systems are unpredictable, which is why traditional models can fall short. For example, many central banks use mathematical models to understand how the economy responds to policy decisions. However, these models are often based on the assumption that people always act rationally, considering every piece of information before making a decision. In reality, people often make decisions based on incomplete data, gut instinct, or even their current mood—a concept known as bounded rationality.
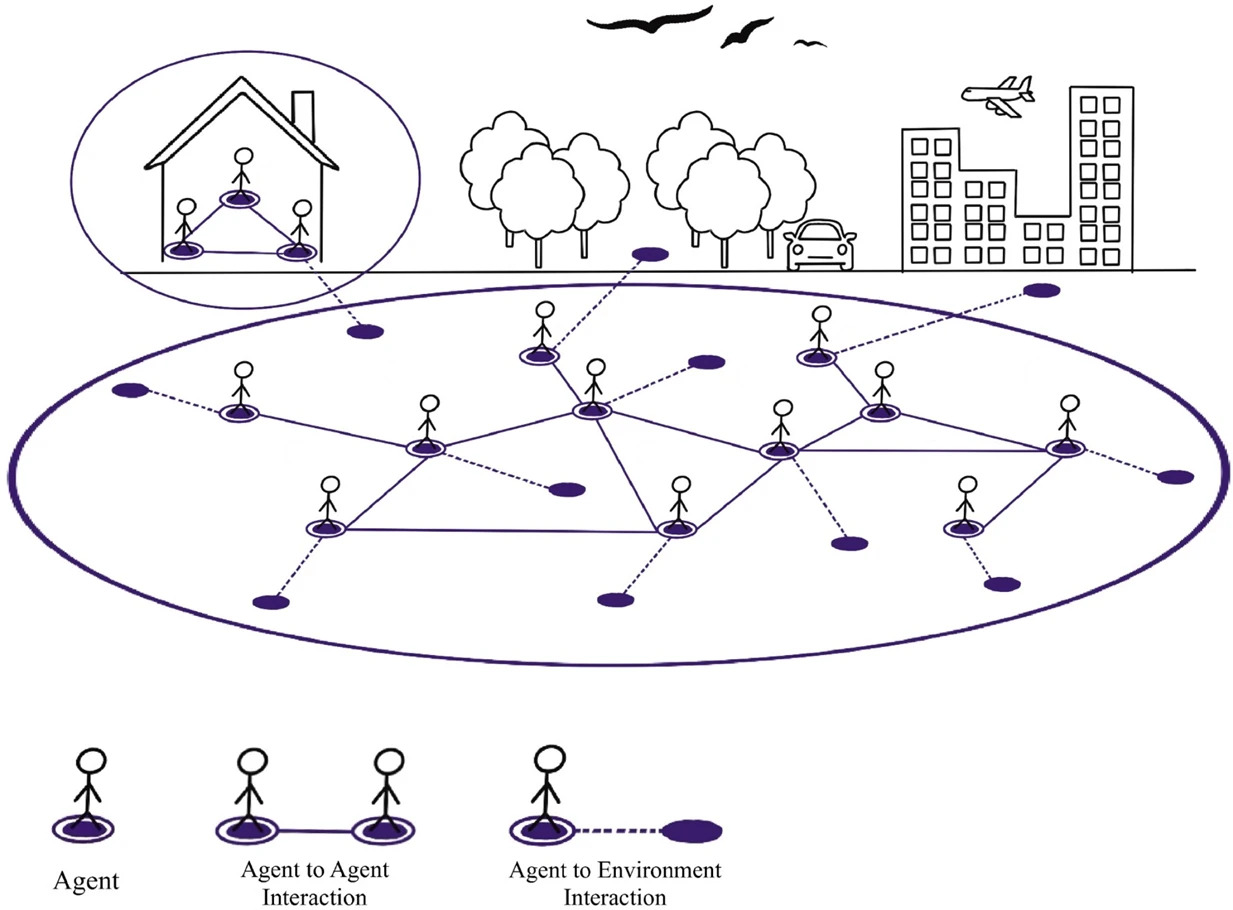
In the late 1980s and early 1990s, researchers began using Agent-Based Models (ABMs) to better understand these complex systems. ABMs allow us to simulate how individuals in a system interact, each with their own set of behaviors and rules. Over the years, with help from deep pools of data and cheap, fast computational power, ABMs have become even more powerful. During the COVID-19 pandemic, ABMs were used to help policymakers decide which sectors of society should reopen and helped analyze how small economies function under central bank control.
At ARLIS, we are developing sophisticated ABMs to address difficult decisions our sponsors face in national security. The Critical Technology Protection Decision Framework (CTP-DF) program enables a decision-making capability that empowers policymakers to understand and make informed decisions about how to protect critical technologies to provide a more comprehensive understanding of the potential risks and rewards of technology protection. Decisions about technology protection are often influenced by subjective views or political pressures. Without objective, data-driven analysis, these decisions can have consequences that can stifle innovation and impair effective technology protection.
Our work on CTP-DF shines through with our Innovation Policy Lab to address these potential consequences. We can test potential policy impacts in a controlled, simulated environment. By using data and rigorous analysis, this lab can provide insights that help policymakers understand the long-term effects of their decisions. ARLIS is helping to ensure that these policies minimize risks and maximize desired effects on the U.S. innovation ecosystem.
The viewpoints, opinions, and conclusions expressed in this article are those of the author(s) and do not necessarily reflect the policies, positions, or endorsements of ARLIS, UMD, or any affiliated organizations. The content provided is for informational purposes only and should not be construed as representing the views of any funding agency.